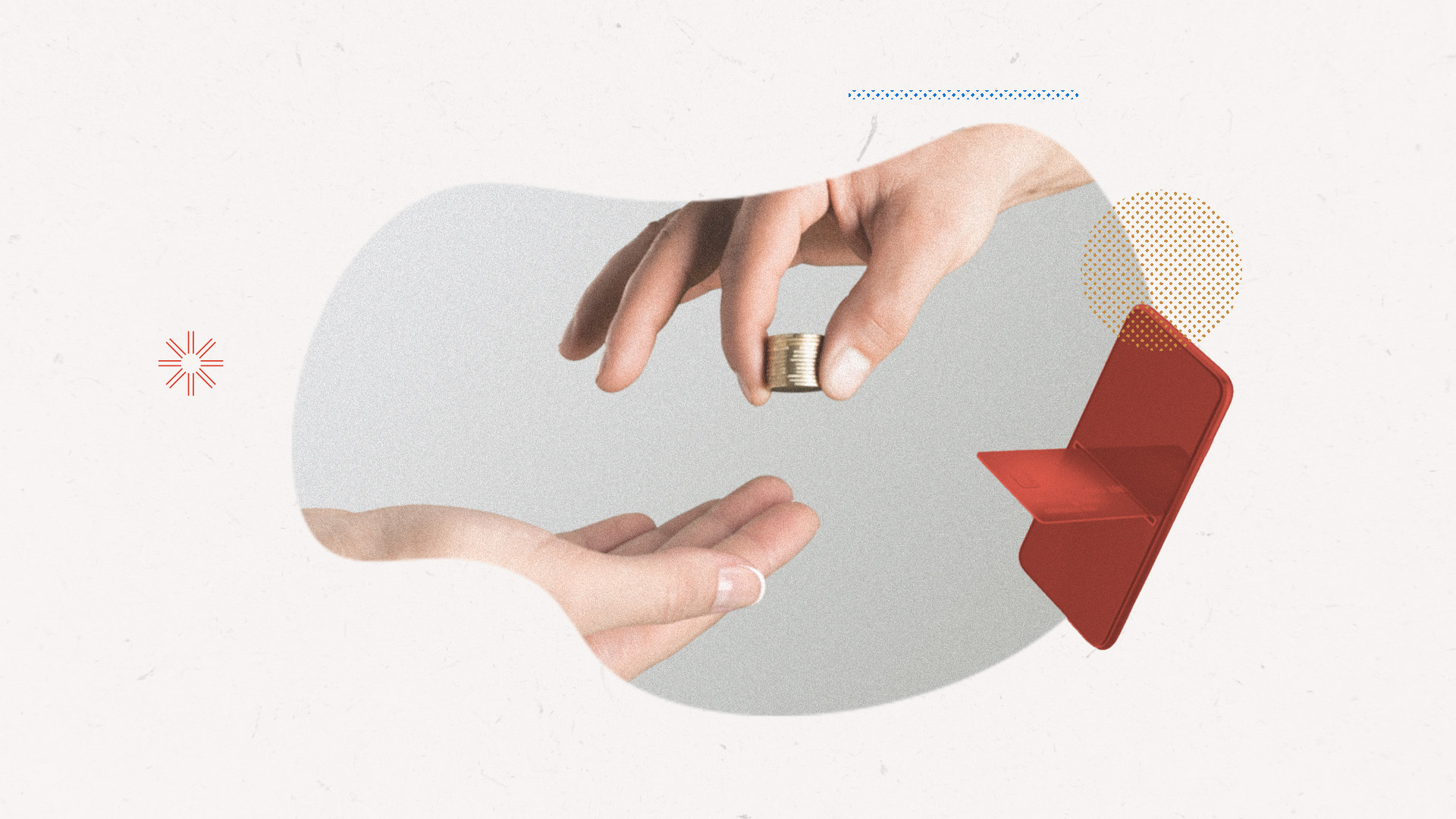
As bank regulators once again question the fairness of artificial intelligence in loan decisions, online lenders and FinTechs agree that the technology should be used carefully, transparently and with tests for bias and disparate impact.
Rohit Chopra, director of the Consumer Financial Protection Bureau, warned recently that the artificial intelligence in loan decisions could lead to illegal discrimination. Online lenders say that with the right safeguards in place and good motives, loan decisions made using AI are more fair than those of traditional underwriting systems. Plus, tech-enabled loans expand access to credit, they claim.
The fresh regulatory scrutiny from a Democratic administration could bring new rules and enforcement actions that affect the FinTechs and banks that use AI in lending decisions, and the industry is fine with that.
"AI is not perfect," said Jason Altieri, general counsel and chief compliance officer at the online lender Happy Money and former general counsel at LendingClub. "If you have humans designing something, bias will creep in. It just will. It may be by the designers or it may be the limitations of the data that they're using."
Yet Altieri says AI is less biased than human loan officers.
"The way it was done for years was you'd walk in, you'd ask your banker for a loan, and they would look at you and with absolute bias say, Do I know you, do you have an account here and do you look like me?" Altieri said. "That's not how we make loans anymore. In 2009, when I joined LendingClub, it was, we don't even see you, we don't even know you, you were just a series of data points. That made it better for everybody." AI also allows the use of nontraditional data like how current a borrower is on their rent and utility payments, he said.
"I absolutely think this is the way it needs to go," Altieri said.
Upstart and the CFPB
This debate started five years ago, when Richard Cordray was the CFPB director and online lenders that use AI in their underwriting, like LendingClub, Prosper, OnDeck and Upstart, were growing fast. The bureau worked out an agreement with Upstart through which it gave the company a no-action letter (essentially a promise not to bring enforcement action against the company for its use of automated underwriting) in exchange for quarterly data about the lender's loan approvals and denials and its handling of fair-lending rules. The no-action letter was renewed in 2020.
"I would say there hasn't been a significant change in [the no-action letter] process across the last three administrations," said Nat Hoopes, head of public policy and regulatory affairs at Upstart. "It's sharing data - what are the variables in the model, all the information that the CFPB [requests] to poke and prod at it - and getting feedback and being quite transparent on everything we're doing. And then taking their input and iterating to the next phase."
The CFPB gets a lot of transparency out of that process, "not just from Upstart, but about AI in general, and how much can AI benefit borrowers of color, especially," Hoopes said.
In 2019, the CFPB compared the results of Upstart's AI-based loan decision model to a more traditional model and published the results.
"The tested model approves 27% more applicants than the traditional model, and yields 16% lower average [annual percentage rates] for approved loans," the CFPB wrote. "This reported expansion of credit access reflected in the results provided occurs across all tested race, ethnicity, and sex segments resulting in the tested model increasing acceptance rates by 23% to 29% and decreasing average APRs by 15-17%."
Under the Chopra-led CFPB, "You have to be prepared for more scrutiny," Hoopes said. "You really need to be checking your algorithms and making sure that they comply with Equal Credit Opportunity Act and disparate impact rules and that you're doing all that testing the right way. But I also see an enormous amount of focus [from Chopra] on any legacy provider that has a de facto monopoly, where the consumer isn't getting a good deal."
This time around, regulators are more educated, in part because of the CFPB's work with Upstart.
"The machine learning analysts that they've engaged with are very familiar with this," said Teddy Flo, general counsel at Zest AI, a former online lender that now provides AI-based lending software to banks. "They really leveled up their understanding of the technology and are now asking much more granular questions: Are you doing disparate-impact testing? Are you doing disparate-treatment testing? Which explainability method are you using?" Regulators are also looking more closely at the data sources used to train the models, and if they are compliant, he said.
'Algorithms can never be free of bias'
Chopra has said that algorithms can never be free of bias and that they may result in credit determinations that are unfair to consumers.
AI lending technology vendors agree that there are dangers to using AI in lending decisions.
"Left to their own devices, there's no question that these algorithms will discriminate when they encounter a subpopulation that is not well represented in the data," said Kareem Saleh, founder and CEO of FairPlay, a developer of software that tests loan decisions for signs of bias, disparate impact and discrimination. People of color, for instance, have historically been excluded from the lending system, and therefore less data is available about how their loans perform.
"When you encounter those folks, having very little data about them, the algorithm will necessarily assume that they're riskier," Saleh said.
The algorithms have to be tested to see if their outcomes are fair, Saleh said. But there's also an opportunity for an algorithm to pick up on clues that disadvantaged people resemble good applicants who were approved.
FairPlay's software can take a second look at declined applicants using information about underrepresented populations. For instance, if the model encounters someone with inconsistent employment, rather than automatically assuming that they're riskier, it can recognize that women sometimes take career breaks, and that doesn't make them irresponsible borrowers.
When FairPlay reviews loan decisions, "We find that 25% to 33% of the time, the highest-scoring people of color and women that get declined would have performed as well as the riskiest white men that most lenders approve," Saleh said.
This all assumes the lenders using AI have worthy intentions, which is not always the case.
"There are actors out there that are not doing it well, that are not explaining their decisions with accurate methods and that are not focused sufficiently on fair lending," Flo of Zest AI said. That's why he and others agree with the principles the CFPB is articulating.
"It's not the AI, it's the intentionality in reducing the racial disparity that's important," said Chi Chi Wu, staff attorney at the National Consumer Law Center.
Calling AI a black box
Lorelei Salas, assistant director for supervision policy at the CFPB, warned in a recent blog post that the bureau plans "to more closely monitor the use of algorithmic decision tools, given that they are often 'black boxes' with little transparency."
This is not a new criticism.
Jason Altieri was general counsel at LendingClub in 2014, when the Securities and Exchange Commission asked the company to include its lending algorithms in the S-1 document it was preparing before its initial public offering.
Altieri had two objections: First, no one would understand it. Second, "that's like asking Coke to put the recipe for Coke out into the public. You just can't do that. You've got to respect that these companies are there to make credit available on better terms to broader swaths of folks, but they are a business. And so you're going to have to tolerate not knowing how it works, but then trust that the [fair-lending] testing takes care of it."
Transparency comes from testing individual variables and clusters of variables to make sure that there's no proxy for a protected class, Hoopes said. And all model outputs need to be run through fair-lending tests and disparate-impact tests.
"You need to achieve acceptable fair-lending results, but then you also should be trying to expand access to credit," Hoopes said. "According to the Urban Institute, only 20% of Black borrowers have a credit score over 700, compared to 50% of white borrowers. So if you rely on a traditional underwriting model with a 700 credit score cut-off, you are denying a chance to so many minority borrowers who have never ever defaulted on loans, yet have somewhat lower scores."
Companies that use AI in lending today believe the real black boxes are the traditional models banks use to calculate credit scores and weigh credit decisions.
When Zest produces a model, Flo said, it comes with a 50- to 100-page report explaining every feature in the model, how it works, and how important it is to every decision. It also provides a 20-page fair-lending report that talks about each variable's contribution to disparate impact.
"It verifies there's no disparate treatment," Flo said. "That's what responsible machine learning practitioners are putting in the hands of lenders. Whereas FICO gives you a three-digit number and one of 20 codes explaining the decision, with no transparency into how they got there." FICO did not respond to a request for comment by press time.
One company working to root out bias in its models is Happy Money in Tustin, California, an online lending partner to several banks and credit unions. The company is implementing Fairplay's software alongside its lending algorithms, and, like its peers, is testing regularly for bias. Most lenders today test their loan decisions for possible fair-lending violations on a quarterly basis, Altieri said.
"I can take a potential model, run it through and be able to see real-time if I inadvertently tripped over something where people of color are being declined, and if so, not roll it out and redesign it," Altieri said. "That's the key piece here: very fast feedback. Historically, it would take a long time to see what the actual effects of a model were."
This article was written by Penny Crosman from National Mortgage News and was legally licensed through the Industry Dive Content Marketplace. Please direct all licensing questions to legal@industrydive.com.